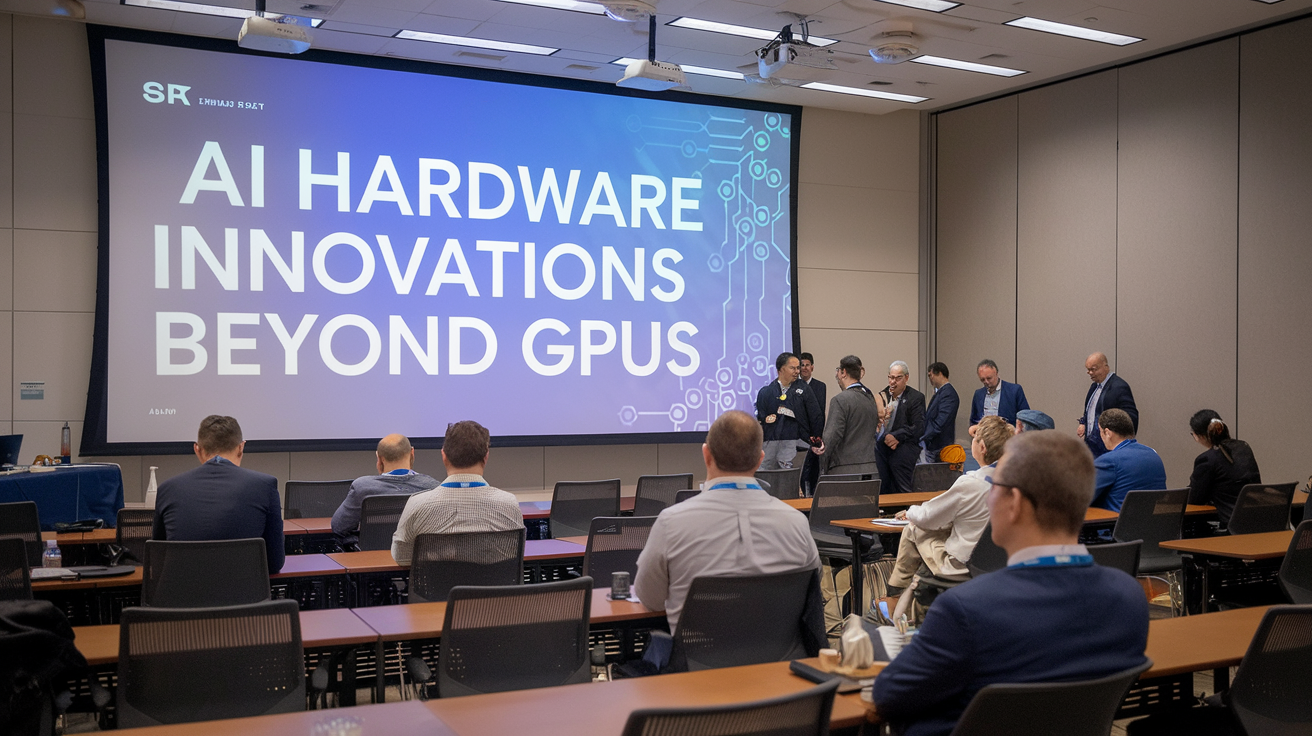
AI Hardware Innovations Beyond GPUs: What's Next?
Oh, how the world of AI has changed — none too surprising; we are looking beyond GPUs into newer silicon. These require more computational power and efficiency (hardware). Read about what we can expect next when it comes to AI hardware at the AI Hardware and Edge AI Summit.
Bigkeynote, with a focus on Tensor Processing Units (TPUs): These chips are built for machine learning and perform much better than GPUs in certain tasks. Tech giants like Google are now making these units.
We also talked about neuromorphic chips. These chips work the same way the rain brainsult in AI, which is highly energy efficient and fundamentally structured differently, resulting in better mobile AI.
There has also been the merging of quantum computing and AI. The result might allow AI to run faster and open up new chapters in machine learning. Other new concepts, such as optical computing and in-memory computing, are also reshaping the way we think about AI.
Bigkeynote, with a focus on Tensor Processing Units (TPUs): These chips are built for machine learning and perform much better than GPUs in certain tasks. Tech giants like Google are now making these units.
We also talked about neuromorphic chips. These chips work the same way the rain brainsult in AI, which is highly energy efficient and fundamentally structured differently, resulting in better mobile AI.
There has also been the merging of quantum computing and AI. The result might allow AI to run faster and open up new chapters in machine learning. Other new concepts, such as optical computing and in-memory computing, are also reshaping the way we think about AI.

Key Takeaways
- Tensor Processing Units (TPUs) are emerging as specialized chips for accelerating machine learning workloads, offering superior performance and efficiency compared to GPUs.
- Neuromorphic chips are mimicking the architecture of the human brain, enabling energy-efficient and event-driven AI computations.
- Quantum computing and AI are converging, with the potential to unlock new frontiers in machine learning through the unique properties of quantum mechanics.
- Optical computing and in-memory computing are innovative hardware architectures that are challenging the traditional computing paradigms.
- Spiking Neural Networks (SNNs) and analog AI accelerators are exploring alternative approaches to AI computations, inspired by biological neural dynamics.
Emergence of Tensor Processing Units (TPUs)
As the need for fast and efficient machine learning grows, companies like Google have created their own AI chips called Tensor Processing Units (TPUs)2. These chips are made to do well at tensor operations, which are key in AI and deep learning. They offer better performance and use less energy than traditional GPUs for some tasks.
Google's Custom AI Chips for Machine Learning Workloads
Google's TPUs show the company's dedication to AI hardware innovation2. These custom chips are made to handle the tough tasks of training and using big machine learning models. This lets Google use its AI progress at a hardware level.
TPUs Designed for Efficient and Accelerated Tensor Operations
TPUs stand out because of their special design for fast and efficient tensor operations2. They are great for tasks like neural network inference, where quick data processing and low power use are key. As AI and deep learning grow, TPUs will play a big role in the most advanced and efficient AI apps.
"With TPUs, we can take an AI model trained on the powerful TensorFlow machine learning framework and deploy it on custom hardware that's optimized for that model's specific needs, resulting in huge performance and efficiency gains."
- Urs Hölzle, Senior Vice President of Technical Infrastructure at Google
Neuromorphic Chips: Mimicking the Brain's Architecture
Artificial intelligence is growing fast, and a new type of chip is leading the way. These chips, called neuromorphic chips, try to work like our brains. They use special networks and computing methods that are more energy-efficient than old systems3.
Big names like Intel and IBM are working hard on these chips. They want to make AI work better and use less power. Researchers in Bengaluru have made a big leap by creating a chip with 16,500 states, way more than the usual two4.
These chips use a lot less energy than old AI systems. This is great because big AI models need a lot of power. A team in Bengaluru showed how these chips can work wonders with less energy, even making famous images4.
Neuromorphic chips could change many fields, like AI, finance, and healthcare. They can handle big data fast and use less energy4. This means AI can learn and work faster, which is really good for many areas4.
As these chips get better, we'll see AI that can do more without using a lot of power or space4. Making chips like these is a big step towards AI that works like our brains5.
"Neuromorphic systems use significantly less energy compared to traditional deep learning models, which require massive amounts of computational power, leading to high energy consumption, especially for large models like those used in natural language processing."
- Spiking neural networks and neuromorphic hardware platforms are getting wide attention and are being applied to relevant problems using Machine Learning5.
- An unprecedented number of neuromorphic systems are supported by the Neuromorphic Intermediate Representation (NIR), which enables interoperability between platforms and improves accessibility to multiple neuromorphic technologies5.
- Various configurable neuromorphic systems have been developed over the last decades, ranging from analog/mixed-signal to processor-based solutions5.
- PyNN is the most widespread common interface to neuromorphic hardware, supporting different neural simulators and systems, while NeuroML provides a serializable set of biological cell and network models emphasizing computational correctness5.
- Fugu provides an SNN graph as an intermediate representation for neuromorphic simulators or hardware compilers, and Lava simplifies programming of the neuromorphic chip Loihi but requires synchronization messages5.
- Nengo is a mature library for brain simulation and deep learning-inspired spiking networks connected to several neuromorphic systems, and several frameworks have been developed to design neuromorphic algorithms, including the SNN-Toolbox, NxTF, and hxtorch.snn5.
- Zhang et al. have developed an abstraction hierarchy for brain-inspired computing portable across both von Neumann and neuromorphic architectures5.
AI Hardware Innovations Beyond GPUs
The need for AI in many areas has made old computing ways seem outdated. Now, people are looking at new hardware to make AI work better. This new tech aims to solve the problems of old computing methods6.
Exploring Alternative Hardware Architectures for AI
Companies are trying out new ideas like computational storage and analog AI accelerators. These new designs want to make AI work faster by getting closer to the data. This means less time spent moving data around and more time on actual work6.
Astera Labs Inc (NASDAQ:ALAB) saw its revenue jump by 45% from 2022 to 2023, reaching $116 million. This shows how much people want their AI hardware6. Dell Technologies Inc (NYSE:DELL) also saw a big jump, with its AI server sales growing by 80% in a year. This shows the whole industry is moving towards better AI hardware6.
Overcoming Limitations of Traditional Computing Paradigms
The search for new AI hardware is because old ways can't handle today's AI needs. New ideas in how data is handled and stored aim to solve these problems. They hope to make AI work much better6.
As AI keeps getting better, finding new hardware will be key. It will help AI reach its full potential and lead to more progress6. Companies like Astera Labs, Palantir Technologies, and Dell Technologies are at the forefront of this exciting time6.
Quantum Computing and AI: A Promising Fusion
Quantum computing and AI together could change AI hardware forever. Quantum computers use quantum mechanics to solve problems way faster than regular computers7. This could lead to big improvements in AI, especially in optimization, simulation, and learning complex models7.
Experts and companies are working hard to use quantum mechanics to speed up AI. This mix of quantum computing and AI could change how we compute, leading to new discoveries in medicine, finance, and science8.
Harnessing Quantum Mechanics for Accelerated AI Computations
Quantum computers are great at tasks that regular computers can't handle, like big number factoring and complex system simulation7. They use quantum mechanics to work super fast and efficiently7. This could greatly help in making AI algorithms better, faster, and more accurate.
"The fusion of quantum computing and AI holds immense potential for revolutionizing the field of AI hardware."
As quantum computing gets better, scientists are finding ways to mix it with AI7. This mix could lead to huge advances in drug discovery, material science, and climate modeling8.

But, there are big challenges ahead. We need to think about ethics, how to understand the AI, and avoid biases in the data7. Still, the idea of quantum computing and AI together is very exciting. It could change the future of computing and more.
Optical Computing: Unleashing the Power of Light
A new technology is changing the game in AI hardware: optical computing. It uses light to do calculations, making AI faster and more energy-friendly. Photonic processors use light circuits, not electronic ones, to break through old computing limits9.
Light-based computations in optical computing promise better AI processing. It could speed up AI system development. This tech offers faster speeds, less energy use, and better growth than old chips9.
- Optical computing uses photons' speed and low energy for fast calculations.
- Photonic processors can handle lots of data at once, great for AI tasks like deep learning.
- It's also very energy-efficient, which helps with the big energy needs of today's computers.
As AI becomes more important, optical computing is a big chance to explore new areas. It lets researchers and engineers work on AI that's faster, more efficient, and scalable9.
"Optical computing holds the promise of enabling more efficient AI processing and accelerating the development of advanced AI systems."
The growth in optical computing and photonic processors is changing AI and computing. As we keep improving these technologies, the future of AI looks brighter every day9.
In-Memory Computing: Eliminating the Von Neumann Bottleneck
Researchers and tech companies are looking for new ways to make computing faster for AI. They're exploring new hardware that goes beyond the old von Neumann model. In-memory computing is a promising idea. It processes data right in memory, not moving it back and forth like before10.
This method could make computing much faster and use less energy. It gets rid of the slow part of the old model. Companies are working on making in-memory computing work well with AI. This will help data be processed faster in memory for many AI tasks10.
Processing Data Directly Within Memory for Faster Computations
In-memory computing lets data be processed right where it's stored. This skips the slow part of the old model and brings big performance gains. It could change how we do AI computing, making it faster and more efficient for today's AI needs10.
"In-memory computing is a game-changer for AI, as it allows us to process data where it's stored, eliminating the bottleneck associated with the von Neumann architecture."
As we keep improving in-memory computing, we'll see more progress in AI hardware. This will lead to faster, more efficient, and more powerful AI solutions10.

Spiking Neural Networks: Emulating Biological Neural Dynamics
Spiking neural networks (SNNs) are a new way to make AI work like our brains. They use spikes or pulses to talk to each other, unlike old AI that keeps going without stopping5. This makes SNNs use less power, which is great for saving energy10.
Exploring Event-Based Computing for Energy-Efficient AI
SNNs are good at handling information because they work in bursts, not all the time10. This is better than old AI that uses a lot of data. People are working hard to use SNNs for many AI tasks, like seeing pictures and understanding words11.
New chips, like Intel's Loihi, show how SNNs can use much less energy than old computers10. This means we can make AI that works well even when it's far from the internet11.
As AI gets better, SNNs and event-based computing will be key. They help AI use less energy and work like our brains. This opens up new ways for AI to learn and think51011.
Conclusion
AI hardware innovations have changed the game, moving beyond traditional GPUs. We now have tensor processing units (TPUs) and neuromorphic chips that work like the brain. These new tools are set to change how we do AI, making it faster, more efficient, and energy-smart12.
Exploring new hardware like optical and in-memory computing could break through old limits. Spiking neural networks, inspired by the brain, might lead to AI that uses less energy13.
As AI becomes more important, these new tools will be key. They will help us do more with AI, reaching new heights. The future of AI is bright, promising to change many areas of life and expand our knowledge.
FAQ
What are the key AI hardware innovations beyond GPUs that are emerging?
The world of AI hardware is changing fast. New solutions like tensor processing units (TPUs), neuromorphic chips, and quantum computing are coming. We also see optical computing, in-memory computing, and spiking neural networks (SNNs).
What are Tensor Processing Units (TPUs) and how do they benefit AI workloads?
Google made Tensor Processing Units (TPUs) for AI. They're made for machine learning tasks. TPUs are better at tensor operations, which are key for AI and deep learning.
These chips are more efficient and use less energy than GPUs for some AI tasks.
How do neuromorphic chips differ from traditional computing architectures?
Neuromorphic chips try to copy the brain's structure. They use spiking neural networks and event-based computing. This makes them more energy-efficient and good at parallel processing.
Intel and IBM are leading in making these chips.
What are some of the alternative hardware architectures being explored for AI?
As AI gets more complex, new hardware is being made. This includes computational storage and analog AI accelerators. These aim to improve AI processing efficiency beyond traditional computing.
How can quantum computing be leveraged to accelerate AI computations?
Quantum computing and AI together could change AI hardware. Quantum computers are much faster for some tasks. This could help AI in optimization, simulation, and training complex models.
What are the benefits of optical computing for AI applications?
Optical computing uses light for faster, lower-power processing. It could make AI systems more efficient and scalable. This could speed up AI development and processing.
How can in-memory computing address the limitations of the von Neumann architecture?
In-memory computing processes data in memory, not in a separate processor. This can boost performance and cut energy use. It avoids the von Neumann model's bottlenecks.
What are the advantages of spiking neural networks (SNNs) for energy-efficient AI?
Spiking neural networks (SNNs) mimic biological neurons. They use spikes for communication, which is energy-efficient. This could lead to more efficient AI systems.
Tags:
#quantum computing
# neuromorphic chips
# hardware
# GPUs
# AI Hardware